Estimating the current market value of used cars increases profitabilityAutomotive Industry
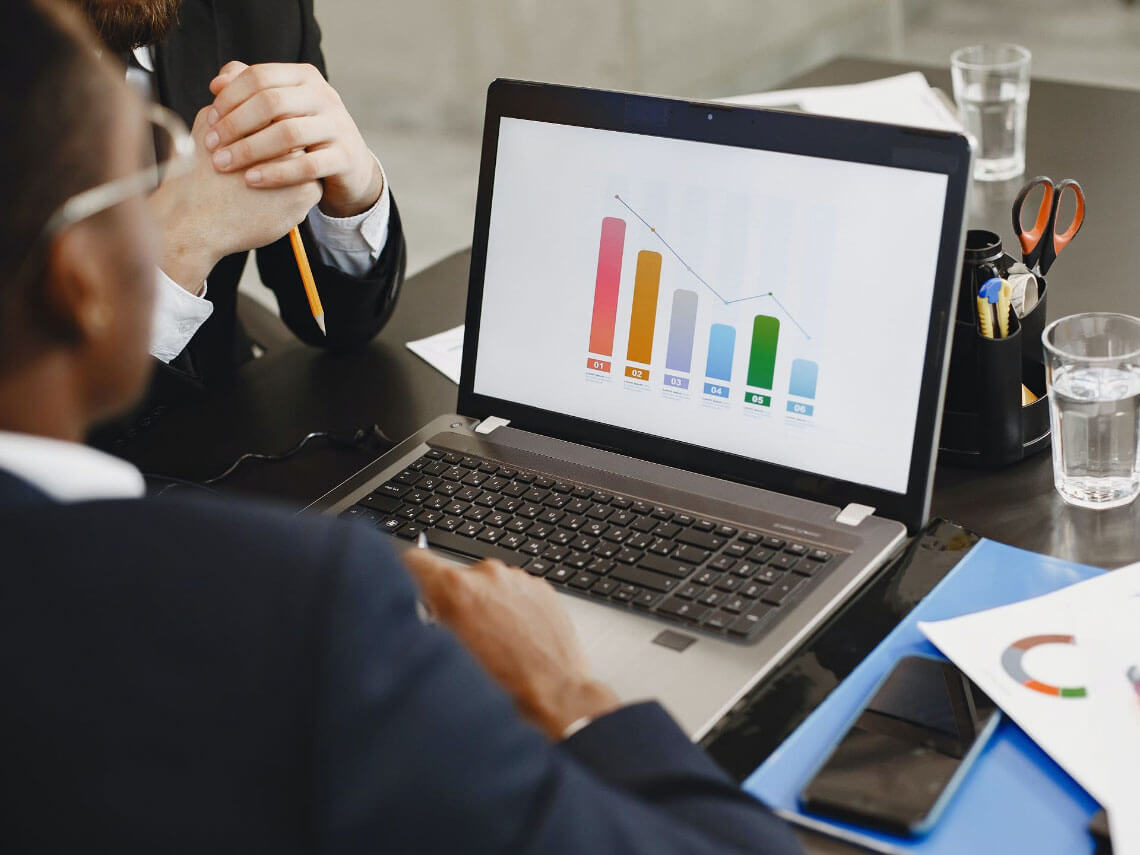
The internal pricing matrix used by the sales staff was built by the pricing team at the headquarters and major revisions were made just once a year. While the branches had some discretion for the range of the prices, it was observed that about 8% of the transactions were grossly undervalued.
FOYI was involved to craft an on-demand car valuation process so that the pricing team can revise the prices more often.
Challenge
The used-car sales customer proposed a project scope with the task of building a car price prediction application. The idea was to use this application to generate on-demand pricing based on the recent changes in the market pricing and therefore allow the pricing team to revise the pricing matrix more frequently so that the prices reflect the current market conditions.
To this extent , FOYI proposed a 2 day discovery workshop to understand the current state of the data and estimate what the project timelines, dependencies and the cost. As part of the 2 day discovery workshop, the following challenges were uncovered.
1. Vintage and limited edition cars were valued very high.
Age of the car is key attribute of car valuation i.e older the car, lower the value. However, a 30 year old car is valued more than a 5 year old car on account of a special value for vintage and other reasons. Such data cannot be used to train a machine learning model.
2. The value at auctions had a wider range compared to buy-now.
Broadly speaking, there were two channels of sale at this customer namely, auction and buy now. The customer prefers to put the cars in auction first and when they are not sold for a few months, they put a sticker price and move it to buy-now. Since the price in auction varies drastically, sometimes as much as 80% higher than the ask price, this data cannot be used for training the machine learning model.
3. The value at 2 biggest cities were at least 8% more than the rest.
The stock and the prices were different between the branches across the country. Therefore, the influence of the branches cannot be ignored while training the machine learning model.
Approach
The challenges identified as part of the 2 day discovery workshop helped FOYI propose the scope of the project and the approach to solving the problem as follows.
1. The value of the vintage and special/limited edition cars was more subjective and therefore such transactions (around 3% of the total transactions) was excluded from this project.
2. The value at the auction was also dependent on the bidding audience and was more subjective given the emotional buying behavior at the auctions. Therefore, auction related transactions(around 27% of total transactions) were excluded from the project.
3. Some branches were more similar to each other than the others based on the valuations of similar cars. Therefore, a branch segmentation model needs to be built and fed into the price prediction model.
Outcome
The 3 key deliverables of this approach and their benefits are as follows.
1. Insights dashboard on the historical sales data from 2 day discovery workshop.
Benefit: An on-demand report that provided visibility on the changes in the market valuation and helped in identifying the right time to revise their pricing matrix
2. Grouping branches into meaningful segments from the segmentation model.
Benefit: The segmentation of the branches helped the sales teams to get insights into sales targets for the branches.
3. An on-demand price prediction process.
Benefit: A well documented price prediction process that the internal IT team was trained on enabled the business to revise the pricing matrix faster and more frequently.
Contact us for an introductory call to understand how we can help you.